Assessing Nigeria’s Data Protection Readiness in the Age of Large Language Models (LLMS). By Imoter Kelvin Kpengwa Winner Lawyers Category of 5th Edition of Adavize Alao Essay Competition
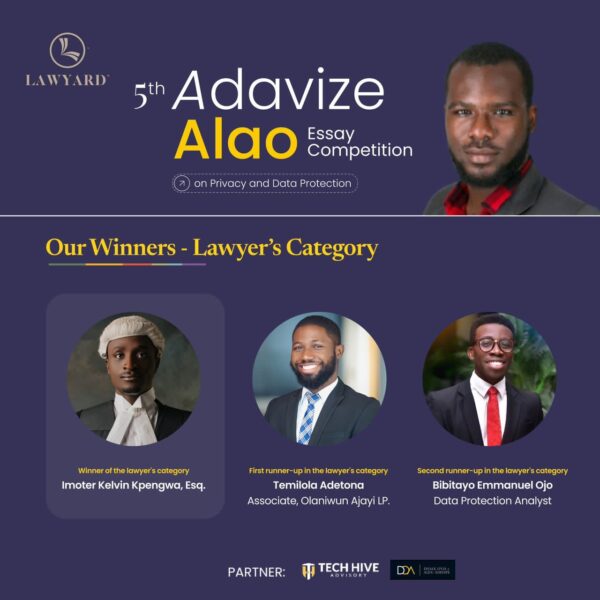
Lawyard is a legal media and services platform that provides…
1. Introduction.
The world has witnessed a rapid transformation in the digital landscape in recent years, marked by the emergence of cutting-edge technologies like Artificial Intelligence (AI). One of the most popular applications of this technology is Large Language Models (LLMs), a subset of Natural Language Processing (NLP). LLMs, such as OpenAI’s GPT-31 and Google Bard, have revolutionized how we interact with data and information. They have become lifesavers for professionals in different fields, from medicine to research and art. The excellence of this technology begs the question: How can LLMs give such intelligent and human-like responses to queries? The simple answer is data. These systems are fed with tons of data to help them generate intelligent and human-like responses to user queries. In fact, the larger the data LLM is fed, the more potent it becomes. There is no doubt that where such a large volume of data is involved, the issue of data protection will arise.
This article will look at LLMs, how they work, and the data concerns raised by their use. Then, it will examine the data protection framework in Nigeria vis-à-vis other jurisdictions to assess her readiness to adapt to this global phenomenon.
2. Understanding LLMs.
LLMs are foundation models that use AI, deep learning, and massive data sets, including websites, articles, and books, to generate text, translate between languages, and write many types of content. LLMs need to meet the user where he is to be considered adequate. They must understand language, its many contexts, nuances, and rare phenomena. LLMs must also be able to perform tasks beyond the scope of their training. This is why all LLM training mechanisms involve exposure to massive datasets.While the definition of “large” has been increasing exponentially, these models are typically trained on datasets large enough to include nearly everything written on the internet over a significant time.
For example, in June 2020, OpenAI released GPT-3, a 175 billion-parameter model allegedly trained on 570GB of data scraped from the internet that generated text and code with short written prompts. In 2021, NVIDIA and Microsoft developed Megatron-Turing Natural Language Generation 530B, one of the world’s most extensive reading comprehension and natural language inference models, with 530 billion parameters.
LLMs process the datasets through unsupervised learning, meaning they process the data without specific instructions. During this process, the LLM’s AI algorithm can learn the meaning of words, the relationships between words, and how to distinguish words based on context. The enormous datasets used in training LLMs are what makes them revolutionary. Their uses extend far beyond the realm of language to the world of science. They can be used to write computer codes and even to develop vaccines. The application of LLM technology is limitless, and we are only now scratching the surface.
3. LLMs and Data Privacy.
As already stated, LLMs raise many concerns regarding data protection. The first data privacy concern LLMs raise is whether the models’ developers fall into the traditional data controller or data processor categories. A data controller determines the purposes for which and the means by which personal data is processed, while a data processor processes personal data on behalf of a data controller. Going by these definitions, LLM developers are data controllers in relation to the data they scavenge but are data processors where other entities use them to process their data.
LLMs also raise concerns regarding what kind of data is being collected and used to train LLMs and how long the data is stored. To answer the first question, LLMs are said to be trained with publicly available data, some of which may be private data. This is somewhat vague and implies that private data that became public data due to data breaches would be used to train these models. Social networking sites like Facebook, Reddit, Quora, YouTube, and Medium have been cited for training LLMs. However, the source of most of the data used in training these LLMs remains unknown. In its 2022 report, Language Model for Dialogue Applications (LaMDA), used to train Google Bard, vaguely alluded to 50% of its scraped data as ‘dialogs data from public forums’ without stating which forums it sourced data from. The lack of transparency regarding the source of the data used in training LLMs is a violation of data privacy in some jurisdictions. For example, under Article 14 of the General Data
Protection Regulation (GDPR), data subjects whose data is openly sourced have the right to know what data is being collected and why. In line with Article 14 of the GDPR, the Polish Data Protection Authority (UODO) imposed a fine of about 220,000 Euros on Bisnode, a data aggregation company headquartered in Sweden, for failing to inform data subjects about how it processed their personal data.
Given that developers of LLMs are not forthcoming with the specific sources of the data used to train their models, it is nearly impossible for data subjects to know if their personal data has been used to train the models. Even though the terms of use of Facebook, LinkedIn, and Twitter prohibit web scraping, it remains unknown whether or not they are used to train LLMs regardless, and if yes, the extent of personal data used. Indeed, the whole point of LLMs is to give intelligent human-like responses, and people use sites like the above to express the different contexts and connotations of language, making them appealing to LLM training.
Apart from data sourced from the internet, developers also use end-users’ personal data, like profile information and conversations, to train their LLMs. OpenAI, developers of the popular GPT-3 model, admit using end-user data and their interactions with the platform to improve its performance. The amount and type of data used remain vague, as is the duration it is kept. It is important to note that the end-user can change their settings to prevent their data from being used to improve the system. However, the default setting gives OpenAI the right to use the personal data of its model’s end-users to feed it. While it can be argued that OpenAI fulfills the GDPR’s duty to inform data subjects of the use of their personal data here, there is no doubt that the transparency and duration requirements remain unmet. Additionally, recent research showed that while LLMs are helpful for taking notes during eye surgery, they usually mix surgery notes with patients’ medical history and symptoms, which is sensitive personal data protected under Article 9 of the GDPR.
In view of the above, there is reasonable cause to believe that LLMs could give rise to profiling because they analyze user data to give personalized responses. This was best exemplified in the Cambridge Analytica scandal, where data from Facebook was used to create psychological voter profiles for the 2016 United States election. With LLM technology, scandals like this will happen on much larger scales with little effort.
Finally, LLMs host tons of data, making them susceptible to cyberattacks and subject to the exposure of sensitive information. OpenAI’s ChatGPT was recently the subject of the latter when it announced that ChatGPT’s chart source code, Redis, used to store user interactions per session, had a bug that caused specific data leakage. This led to users having access to the chat conversations of other users and the exposure of payment-related information of some premium users.
4. Accessing Nigeria’s Data Protection Readiness in the Age of LLMs.
The principal legislation regulating data protection in Nigeria is the Nigeria Data Protection Act of 2023 (NDPA). The NDPA established the Nigeria Data Protection Commission (NDPC) as the primary data protection authority in Nigeria. It also retained the Nigeria Data Protection Regulation 2019 (as amended) (NDPR) developed by the National Information Technology Development Agency (NITDA) pursuant to the NITDA Act 2007. Thus, the NDPA and the NDPR are to operate conjunctively, although the former shall prevail where there is conflict.
The NDPA, like the GDPR, gives data subjects the right to be informed about the use and retention period of the personal data collected. It also provides that the data subject must have given and not withdrawn consent for the data to be used. According to the law, consent must be affirmative and not based on pre-selected confirmation and may be written, oral, or electronic. However, the legislation seems archaic as it does not consider the peculiarity of LLMs. As stated earlier, LLMs are data controllers and data processors; as such, it can be argued that the above requirements do not specifically apply to them.
Other than the above, the NDPA and NDPR are silent on LLMs and how they work. It remains uncharted territory as far as Nigeria’s data protection laws are concerned. This is the major loophole in data protection in Nigeria today. Even though the above sections can be generalized to tackle data privacy issues arising from LLMs, such decisions would be innately flawed as the legislation did not anticipate their existence.
5. Recommendations.
The trend of Nigerian legislation tailing contemporary issues appears to be the case in LLMs and data protection. Our principal law, the NDPA, appears unprepared to tackle the peculiar data privacy concerns raised by LLMs despite being passed into law recently. To keep up with the global trend, there is a need for Nigeria to create new legislation to tackle the growing AI phenomenon, including LLMs. This appears to be the trend worldwide with the passing of the European Union AI Act on 16 June 2023.21 The legislation would address the data privacy concerns discussed above and give a more robust regulatory framework for the growing AI industry.
For watertight protection of the right to data privacy of Nigerians, the legislation must first put the interests of data subjects above the interests of LLMs. Additionally, the law should:
a. Create an agency to oversee AI and LLM administration by issuing licenses, making periodic regulations, and other ancillary functions to tackle developing issues in the AI industry in Nigeria.
b. Mandate developers to acquire renewable licenses before their LLMs are allowed to run in Nigeria to ensure they adhere to regulatory standards.
c. Ban unrestricted web scraping in Nigeria and mandate developers to publish a comprehensive list of the type and sources of the data used in training their LLMs, including the duration such data is used.
d. Mandate developers to be transparent about the application of the data obtained at the point of training or from end-users and the scope of its use.
e. Create penalties for breach.With the above provisions, the law could address the live issue of data protection in light of LLM technology.
Conclusion.
LLMs have rightfully taken the world by storm. They make work easier for professionals in many fields and have revolutionized vaccine technology. However, LLMs pose a severe data privacy threat with their existence and functionality. Some of these threats and the ways to address them have been discussed above. In curtailing the harm that these models can cause, it is pertinent that lawmakers put the rights of data subjects above the profitability of LLMs. Only then can the data privacy rights of individuals be preserved in today’s data-insatiable economy.
Lawyard is a legal media and services platform that provides enlightenment and access to legal services to members of the public (individuals and businesses) while also availing lawyers of needed information on new trends and resources in various areas of practice.