Assessing Nigeria’s Data Protection Readiness in the Age of Large Language Models (LLMS). By Temilola Adetona, First Runner-up Lawyers Category of 5th Edition of Adavize Alao Essay Competition
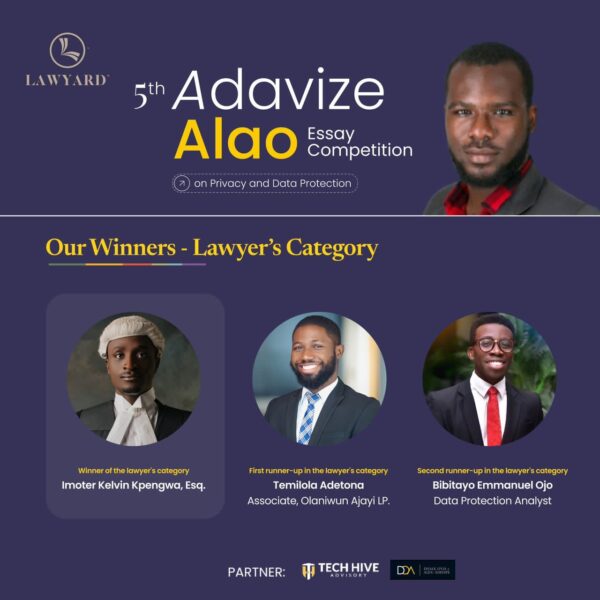
Lawyard is a legal media and services platform that provides…
1.0 Introduction
Data, they say, is the new oil. Although, that description is admittedly a bit of a hyperbole, in today’s increasingly digital world, very few would contest the perceived and actual importance of data. Laudably, many jurisdictions have taken data protection seriously and, therefore, introduced significant policies and laws aimed at regulating data use and protection.
However, technological development evolves faster than we can prepare for it, with the consequent implication being that policy often trails advancement. It is perhaps against this background that Punit Bhatia has remarked that “Data protection is not a goal, it is a journey”. This author reckons this comment to be accurate, and the quest of nations to ensure that the adequacy of their data protection regimes in the era of Large Language Models (LLMs) adequately illustrates how data protection is indeed a journey, an oft unfinished journey. Accordingly, this piece seeks to circumspectly examine whether Nigeria’s data protection regime is indeed fit for purpose in the age of LLMs.
2.0 Conceptual Background
If the foundation be destroyed, what shall this author do? It is, therefore, pertinent at this stage to interrogate, albeit briefly, the key concept that informs the central theme of this piece, viz: LLMs.
An LLM can be described as an artificial intelligence (AI) model trained on great amounts of data subtexts to comprehend and generate human-like language. To achieve this aim, LLMs utilise deep learning techniques, particularly deep neural networks, to process and generate text. Generally, they are characterised as ‘large’ since they are built with a vast number of parameters and demand substantial computational power to train and run successfully.
Put simply, an LLM is a variant of machine learning that is trained on a large dataset with a view to interpreting and generating human like text.
3.0 LLMs and Nigeria’s Data Protection Regime: Ready or Not?
It is crucial at this juncture to consider whether Nigeria’s current data protection framework is equipped to successfully protect the data of Nigerians in the era of machine learning. Thus, this segment provides: an overview of Nigeria’s data protection regime; an assessment of its fitness for purpose; an identification of existent gaps; and recommendations to plug said gaps.
3.1 Overview of Nigeria’s Data Protection Regime
“Relying on the government to protect your privacy is like asking a peeping tom to install your window blinds.” – John Perry Barlow
Whilst Barlow’s sentiments above may resonate with many, the creation of a framework for data protection is a function that is best left to the remit of the government. Accordingly, the Nigerian government has attempted to provide a framework over the years, the evolution of which has ultimately culminated in the Data Protection Act (DPA) 2023 (the Act). Whilst it is impossible to provide a comprehensive overview of the Act in this brief piece, it should be generally noted that the Act applies to the processing of personal data, notwithstanding whether such processing is undertaken by automated means or otherwise. The ambit of the Act is triggered where: (a) the residence or place of operation of the data controller is in Nigeria; (b) the processing of the personal data is undertaken in Nigeria; or (c) although the data controller or processor is domiciled, resident or operating outside Nigeria it undertakes the processing of personal data belonging to a data subject in Nigeria.
The culmination of the foregoing is that where the aforementioned requirements and thresholds are met, the processing of personal data via LLMs falls within the ambit of the Act. However, the question lingers as to whether the regime of the Act is adequately equipped to cater for nuances presented by the advent of LLMs.
3.2 Is Nigeria’s Data Protection Regime Fit for Purpose?
On the face of it, it would appear that the general principles contained in the Act apply to and govern the processing of data via LLMs. The Act demands that those who are categorised as data controllers or processors must: (a) engage in a ‘fair, lawful, and transparent’ processing of data; (b) ensure that data is only collected for ‘specific, explicit and legitimate’ purposes (purpose limitation); (c) in light of the purpose, only collect data that is ‘relevant, necessary and adequate’ (data minimisation); (d) not hold the data longer than necessary (storage limitation); and (e) ensure the confidentiality, integrity, and security of data collected. Furthermore, the Act (x) imposes a duty of care on the data controllers and processors when processing personal data; (y) requires them to demonstrate accountability; and (z) explicitly sets out the six lawful bases for processing personal data.
Although AI and LLMs are not specifically mentioned in the Act, entities who operate LLMs are bound to comply with these internationally accepted principles in the operation of the models, thus creating a base framework for the regulation of data processing by LLMs in Nigeria. However, the nuanced nature of LLMs creates a tension between these traditional principles and the evolving data processing activities of LLMs which warrants specific consideration.
3.2 Nuances, Gaps, and Limitations
As stated earlier, the operation of LLMs is predicated on training the language model on large volumes of data. The sheer volume of data required to be fed into the LLMs is the principal precursor of privacy and data protection concerns especially as regards the nature of the data supplied to the LLM, data security, and the length of time for storing such data. This operational model of LLMs discloses certain nuances and therefore reveals some gaps in the present data protection framework which must be plugged. Some of these include:
a. Application of the principles: Some of the most fundamental principles of direct data collection are that: (i) the data is collected for a specific purpose; (ii) the data subject is notified of the lawful basis for processing; and (iii) the data subject is informed of the recipients or categories of recipients of the processed data. One wonders if LLMs can duly satisfy these principles given that there will likely be significant downstream uses which may not remain within the scope of the stated purpose and the range of potential recipients may be too large to conceive or even specify. How effective then is the consent of the data subject where the use to which the processed data is put exceeds the originally agreed scope?
b. Use of publicly available data: The data upon which the LLMs are trained are often obtained from a wide variety of sources including the internet. This may, therefore, include information made available by individuals themselves on websites, blogs, social media, etc, although without any intention that such information is to be used by LLMs for whatever purpose. Where personal data is collected indirectly the data controller is required to inform the data subject of the lawful basis for processing, the proposed recipients of the processed data, retention period, and the identity of and means of communication with the data controller. This information may be provided in a clear and accessible privacy policy. Further, the Act also provides an exception in that the data subject may not be informed where providing the information would involve disproportionate effort or expenses for the data controller. It is unclear how the privacy policy rule helps LLMs with regards to data fetched indirectly from sources such as social media pages. For instance, if the LLM is trained on data fetched from social media, it is unclear how the LLMs general privacy policy may be communicated to all individuals concerned. On the other hand, there remains the possibility that the LLMs may opt to rely on the disproportionate effort or expenses exception.
c. Data security:
There are also concerns that the large volumes of personal data collected, held and processed by LLMs are subject to a real risk of data loss or theft by hackers or other sinister persons who may then extract sensitive personal identifiable information. An indication of this risk occurred recently with ChatGPT when it was reported that a bug was found in the model’s source code which resulted in some data leakage including conversation history and even payment related data. The Act prescribes that where a breach occurs the data controller/processor is to provide notification to the Nigerian Data Protection Commission and the data subject. One wonders if LLMs that process vast volumes of data are equipped to meet this obligation.
d. Right to be forgotten:
The Act confers a number of rights on Nigerians one of which includes the right to request a data controller to erase their data from the database of the LLM. However, we question how this right can be enforced and how LLMs that have been ‘trained’ on the data in question can be made to ‘forget’ the data.
e. International data transfer:
In light of the ubiquitous nature of LLMs, there is a great likelihood of data transfer across border. Whilst the Act does make provision, in principle, for regulating such transfers, procedural constraints exist such as the ability of the regulator to confirm if any data has been transferred across border, where such data has been transferred to, and if the recipient countries meet the adequacy of protection requirements.
3.4 Recommendations
It warrants emphasis that this piece does not in any way pretend to possess solutions to all of the gaps presented by the nuanced interaction of LLMs with the Nigerian data protection regime and the resolution of some of these issues can only be arrived at upon detailed technical consultation amongst regulators and stakeholders in the industry. Notwithstanding, in the immediate, this author presents the following propositions:
a. Regulators may need to consider and clarify the ways in which operators of LLMs may practically attain satisfactory compliance with existing principles under the Act.
b. Given that the Act draws inspiration largely from the GDPR, Nigeria can take a note from the EU’s attempt to introduce a specialised AI Act that regulates the use and operations of machine learning tools such as LLMs. A law or policy in this regard, can attempt, amongst other things, to specifically address the management processes for the unique risks presented by AI powered models.
c. Rules need to be put in place to properly regulate the collection of publicly available data for the use of LLMs. At present, the principles do not envisage data collection at the volume of the LLMs and the exception that exists creates a situation where LLMs can consistently bypass their obligations to notify data subjects by simply claiming the disproportionate effort or expenses exception.
d. Regulators may also consider the imposition of: (a) bespoke obligations to implement more robust data storage and security measures; and (b) more stringent and specific pseudonymisation obligations on the entities that control LLMs to ensure that when data is collected at such mass volumes personal identifiers are removed which reduces risk to data subjects in the event of a data breach.
4.0 Conclusion
This succinct piece has sought to address the central question of the readiness and fitness for purpose of the Nigerian data protection framework in light of the growing presence of LLMs. In so doing, this author has concluded that the Nigerian regime, spearheaded by the Act, does contain traditional data protection principles that apply to LLMs and form an acceptable basic framework for regulation. However, it has also been observed that there are nuances and consequent gaps which must be addressed in order to cater to the sui generis nature of LLMs. It is hoped that with subsequent stakeholder interaction the Nigerian data protection community can arrive at well considered and enduring solutions to risks posed by LLMs. A further hope exists that this is done in reasonable time as the deployment and use of LLMs grows by the day whether or not policy responds quickly. This situation inspires the author to recall the popular hide and seek ‘call sign’, ready or not, here I (LLM) come.
Lawyard is a legal media and services platform that provides enlightenment and access to legal services to members of the public (individuals and businesses) while also availing lawyers of needed information on new trends and resources in various areas of practice.